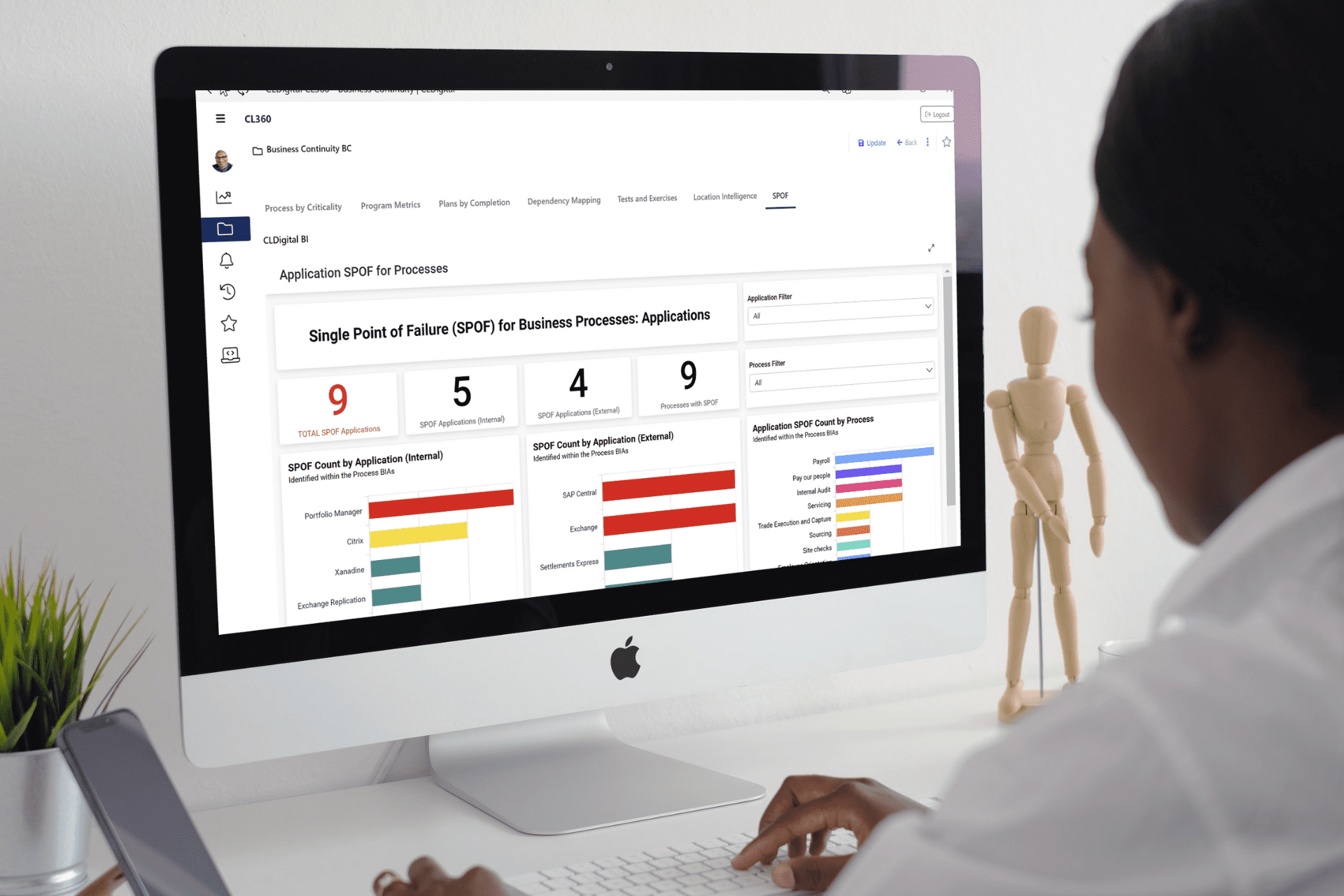
In the ever-evolving landscape of business, Enterprise Risk Management (ERM) has emerged as a cornerstone for sustainable growth and resilience. ERM is not just about mitigating risks; it's about identifying, analyzing, and strategically managing risks in a holistic manner to ensure the long-term success of an organization. In this dynamic environment, the role of data analytics in ERM has become increasingly significant. By leveraging data analytics, businesses can transform their risk management from a reactive to a proactive stance, making informed decisions that align with their strategic objectives.
The integration of data analytics into ERM represents a paradigm shift. It's no longer sufficient to rely solely on traditional risk management methods. The digital age demands a more sophisticated approach, where data-driven insights guide risk assessment and mitigation strategies. This evolution marks a critical step forward in how companies approach risk management, making it a key driver of business strategy and operational efficiency.
Understanding Enterprise Risk Management
Definition and Importance of ERM
Enterprise Risk Management (ERM) is a comprehensive and integrated approach to managing the risks that threaten the assets and earning capacity of a business. It's a discipline that addresses the full spectrum of an organization's risks, including strategic, operational, financial, and compliance risks. ERM is crucial for several reasons:
- Strategic Alignment: It ensures that the management of risks is aligned with the overall strategic objectives of the organization.
- Improved Decision-Making: ERM provides a framework for better decision-making by identifying, assessing, and prioritizing risks.
- Resilience Building: It enhances the organization's resilience to external and internal shocks, ensuring business continuity.
- Value Protection and Creation: Effective risk management protects the organization from losses and creates value by identifying opportunities and optimizing risk-taking.
Key Components of ERM
Effective ERM involves several key components that work together to provide a comprehensive risk management strategy:
- Risk Identification: Recognizing and naming the risks that could potentially affect the organization.
- Risk Assessment: Evaluating the likelihood and impact of these risks.
- Risk Mitigation: Developing strategies to manage and mitigate the identified risks.
- Monitoring and Review: Continuously monitoring the risk environment and reviewing the risk management strategies to ensure they are effective and relevant.
- Communication and Reporting: Ensuring effective communication and reporting mechanisms are in place for risk management activities.
By understanding these components, organizations can develop a robust ERM framework that not only protects them from potential threats but also positions them to capitalize on opportunities that arise from a well-managed risk landscape. The integration of data analytics into each of these components further enhances the effectiveness and precision of ERM, making it an indispensable tool in the modern business world.
The Emergence of Data Analytics in Risk Management
Evolution of Data Analytics in Business
Data analytics has undergone a remarkable evolution in the business world. Historically, decision-making in business was largely intuitive, based on experience and gut feeling. However, the advent of big data and advanced analytics technologies has revolutionized this approach. Businesses now rely on data-driven insights for strategic decision-making. This shift has been fueled by the increasing availability of data, advancements in computing power, and the development of sophisticated analytical tools. Data analytics has enabled businesses to uncover hidden patterns, predict trends, and make more informed decisions.
Integrating Data Analytics into ERM
The integration of data analytics into Enterprise Risk Management (ERM) has been a game-changer. ERM traditionally focused on qualitative assessments and experience-based judgments. The incorporation of data analytics has transformed ERM into a more quantitative and objective discipline. By leveraging data, businesses can now:
- Systematically identify and quantify risks.
- Gain deeper insights into risk exposures.
- Predict potential risk events with greater accuracy.
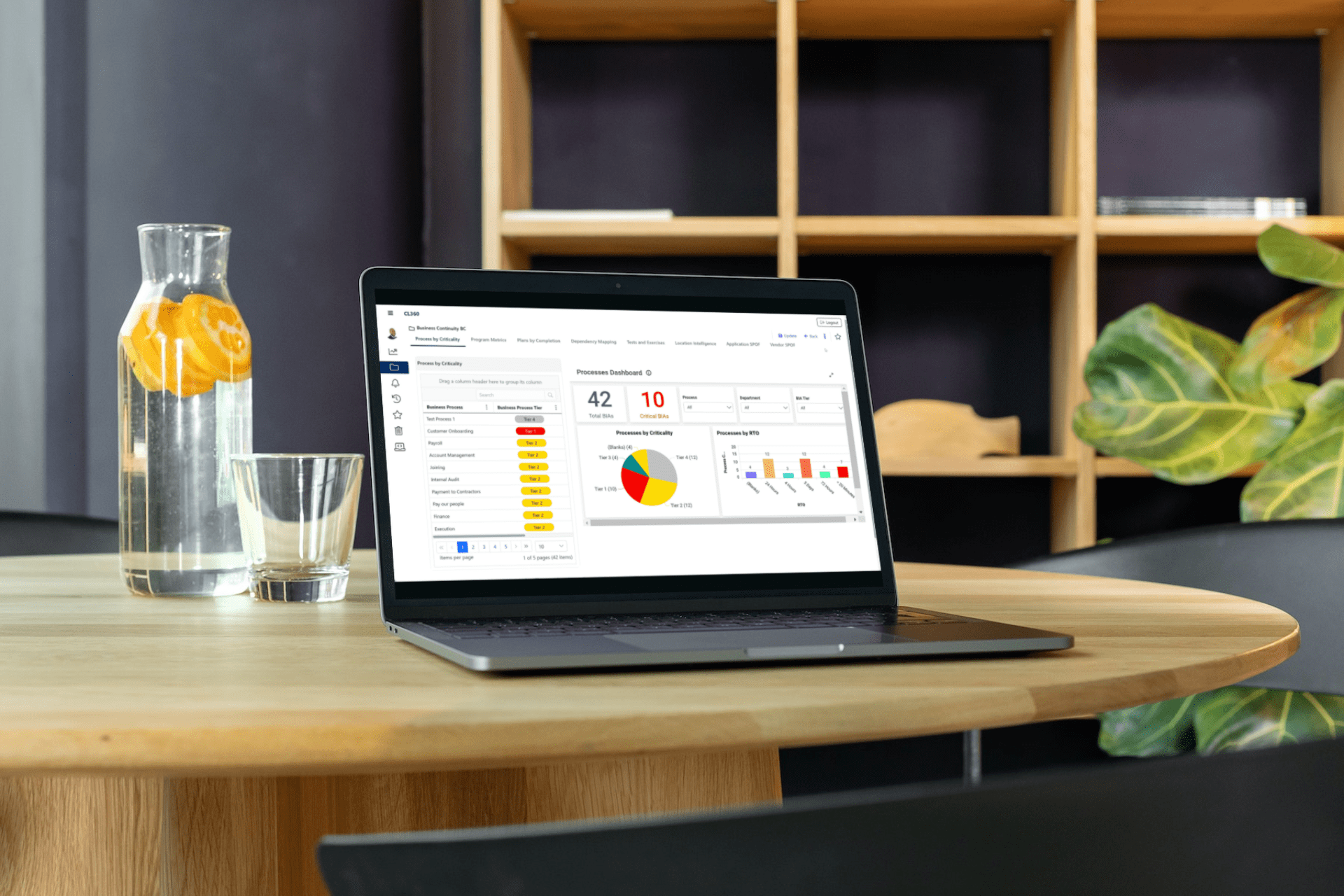
Benefits of Data Analytics in ERM
Enhanced Risk Identification and Assessment
Data analytics significantly enhances the process of risk identification and assessment in several ways:
- Predictive Analysis: Utilizing historical data to predict future risk scenarios.
- Risk Profiling: Analyzing data to create detailed risk profiles, helping in understanding the nature and potential impact of different risks.
- Real-time Monitoring: Continuously monitoring data streams to identify emerging risks promptly.
Improved Risk Mitigation Strategies
Data analytics contributes to the development of more effective risk mitigation strategies by:
- Scenario Analysis: Simulating various risk scenarios to assess the effectiveness of different mitigation strategies.
- Optimization Models: Using data to optimize resource allocation for risk mitigation.
- Tailored Responses: Enabling customized risk responses based on specific risk profiles and business contexts.
Data-Driven Decision Making
The role of analytics in facilitating informed decision-making processes is pivotal. It allows businesses to:
- Make decisions based on empirical evidence rather than intuition.
- Continuously refine strategies based on data-driven feedback.
- Enhance transparency and accountability in the decision-making process.
By harnessing the power of data analytics, ERM becomes a more dynamic, precise, and effective tool in the arsenal of modern businesses, driving them towards greater resilience and strategic success.
Implementing Data Analytics in ERM
Key Steps for Integration
Integrating data analytics into Enterprise Risk Management (ERM) is a strategic process that involves several key steps:
- Assess Current Capabilities: Evaluate the existing ERM framework and identify areas where data analytics can enhance risk management.
- Define Objectives: Clearly define what you aim to achieve with data analytics in ERM, such as improved risk identification, better decision-making, or enhanced risk mitigation.
- Data Collection and Management: Establish a robust system for collecting and managing data. This includes determining data sources, storage, and management practices.
- Select Appropriate Tools and Technologies: Choose analytics tools and technologies that align with your ERM objectives and data infrastructure.
- Integration and Implementation: Integrate chosen tools into the existing ERM framework and implement them in your risk management processes.
- Training and Capacity Building: Train your team to effectively use these tools and understand the insights they provide.
- Continuous Evaluation and Improvement: Regularly evaluate the effectiveness of data analytics in your ERM processes and make necessary improvements.
Tools and Technologies
Various analytics tools and technologies are used in ERM to enhance risk management capabilities:
- Data Management Platforms: For storing and managing large volumes of data.
- Predictive Analytics Tools: To forecast future risk scenarios based on historical data.
- Risk Analysis Software: For quantitative risk assessments and scenario analysis.
- Visualization Tools: To create intuitive dashboards and reports for better understanding and communication of risk data.
- AI and Machine Learning Algorithms: To identify patterns and insights that are not easily discernible through traditional analysis.
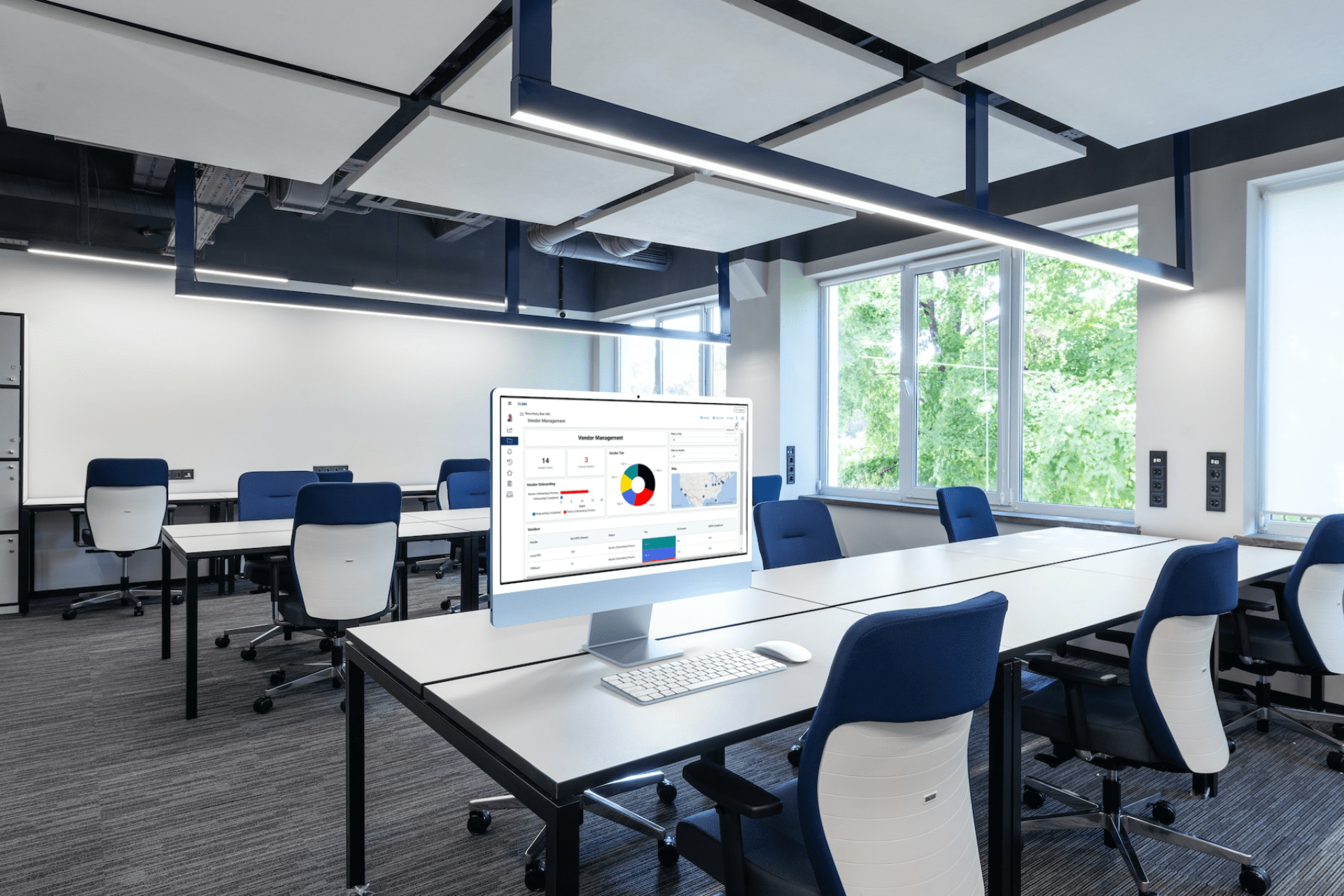
Best Practices for Leveraging Data Analytics in ERM
Ensuring Data Quality and Integrity
High-quality and accurate data is the foundation of effective data analytics in ERM. Best practices include:
- Regularly validating and cleaning data to ensure accuracy.
- Implementing strong data governance policies.
- Ensuring data sources are reliable and relevant to your risk management objectives.
Regular Monitoring and Updating
Continuous monitoring and updating of data and analytics strategies are crucial for maintaining the effectiveness of ERM:
- Regularly review and update risk models and analytics tools to reflect changes in the business environment.
- Monitor data sources for changes that might affect data quality or relevance.
- Stay updated with the latest developments in data analytics and risk management to continually refine your strategies.
Training and Skill Development
Investing in training and skill development is essential for maximizing the benefits of data analytics in ERM:
- Provide ongoing training for staff on new tools and technologies.
- Encourage a culture of continuous learning and improvement in data analytics and risk management.
- Collaborate with external experts or institutions for specialized training and insights.
By following these steps and best practices, organizations can effectively integrate data analytics into their ERM processes, leading to more informed decision-making and enhanced risk management capabilities.
The Future of Data Analytics in ERM
Emerging Trends
The landscape of data analytics in Enterprise Risk Management (ERM) is continuously evolving, with several emerging trends shaping its future:
- Advanced Predictive Analytics: The use of sophisticated models to predict future risks with greater accuracy.
- Integration of Artificial Intelligence (AI) and Machine Learning (ML): These technologies are becoming more prevalent in identifying and assessing risks, offering deeper insights and automating complex processes.
- Real-Time Risk Management: The ability to manage risks in real-time using live data streams is becoming increasingly important.
- Increased Focus on Cybersecurity Risks: As cyber threats grow in complexity, data analytics is playing a crucial role in identifying and mitigating these risks.
- Regulatory Technology (RegTech): The use of technology to manage regulatory compliance, particularly in highly regulated industries.
Preparing for the Future
To stay ahead in the rapidly evolving field of data analytics in ERM, businesses should:
- Invest in Emerging Technologies: Keep abreast of the latest developments in AI, ML, and predictive analytics, and invest in these technologies as they become relevant to ERM.
- Focus on Talent Development: Develop a workforce skilled in data analytics and risk management to leverage these new technologies effectively.
- Adopt a Proactive Approach: Shift from a reactive to a proactive risk management strategy, using data analytics to anticipate and mitigate risks before they materialize.
- Enhance Cybersecurity Measures: Given the increasing cyber threats, prioritize the integration of cybersecurity risk management into the overall ERM strategy.
- Stay Informed on Regulatory Changes: Keep updated on regulatory changes and leverage RegTech to ensure compliance.
Key Takeaways: The Transformative Impact of Data Analytics on ERM
In conclusion, data analytics has significantly transformed the field of Enterprise Risk Management. By enabling more accurate risk identification, assessment, and mitigation, it has become an indispensable tool for modern businesses. The integration of data analytics into ERM processes leads to more informed decision-making, enhanced operational efficiency, and improved risk mitigation strategies.
As we look to the future, the continued evolution of data analytics, with the integration of AI, ML, and real-time data, promises to further enhance the capabilities of ERM. Businesses that invest in these technologies and adapt to these changes will be better positioned to manage risks effectively and maintain a competitive edge in an increasingly complex and uncertain business environment.
The importance of data analytics in enhancing ERM cannot be overstated. It is not just a trend but a fundamental shift in how businesses approach risk management. By embracing this change, organizations can unlock new opportunities for growth and resilience in the face of ever-evolving risks.